Harnessing AI for Smarter Waste Heat Recovery in Industry
- Melchior Krijgsman
- 7 days ago
- 4 min read
Unlocking the Potential of Industrial Waste Heat
In industrial operations, a significant portion of energy input is lost as waste heat, dissipating into the environment without serving any productive purpose. This not only represents a substantial inefficiency but also contributes to increased operational costs and environmental impact.
Traditionally, capturing and utilizing this waste heat has been a complex endeavor, requiring extensive data collection, intricate modeling, and precise system integration. These challenges have often rendered waste heat recovery (WHR) projects economically unfeasible or technically daunting.
The Complexity of Traditional WHR Approaches
Implementing WHR systems involves several intricate steps:
Data Collection: Gathering accurate and comprehensive data on heat sources, temperatures, flow rates, and operational schedules.
System Modeling: Developing detailed thermodynamic models to assess the feasibility of various recovery technologies, such as Organic Rankine Cycles (ORC) or thermoelectric generators.
Economic Analysis: Evaluating the cost-effectiveness of potential solutions, considering factors like capital expenditure, operational costs, and energy prices.
These processes are time-consuming and often rely on fragmented data, leading to delays and increased costs.
Leveraging AI for Enhanced Waste Heat Recovery
Artificial Intelligence (AI) offers transformative potential in streamlining WHR processes:
Data Integration: AI algorithms can efficiently aggregate and analyze data from various sources, providing a holistic view of heat flows and identifying optimal recovery points.
Predictive Modeling: Machine learning models can simulate numerous scenarios rapidly, optimizing system designs for maximum efficiency and cost-effectiveness.
Real-Time Monitoring: AI enables continuous monitoring and adaptive control of WHR systems, ensuring optimal performance under varying operational conditions.
For instance, a study demonstrated that an AI-enhanced tri-level WHR system achieved an overall energy efficiency of 62.3%, adapting effectively to a wide temperature range of 100–800°C (Check the link here: ScienceDirect).
Entropic's Role in Advancing WHR Solutions
Entropic's platform exemplifies the integration of AI in WHR, offering:
Automated Feasibility Studies: Rapid assessment of WHR opportunities, reducing analysis time from weeks to hours.
Optimized System Design: AI-driven simulations to identify the most efficient recovery configurations tailored to specific industrial processes.
Economic Evaluation: Comprehensive cost-benefit analyses, factoring in energy prices, operational costs, and potential incentives.
By streamlining these processes, Entropic enables industries to implement WHR solutions more efficiently, enhancing sustainability and profitability.
Real-World Applications: Where AI Meets Waste Heat Recovery
The integration of AI into waste heat recovery (WHR) is no longer a futuristic concept — it’s actively reshaping how industries design, optimize, and deploy recovery systems. Across sectors, AI is proving instrumental in making WHR both technically viable and economically attractive.
Data Centers: From Digital Exhaust to District Heating
Data centers are among the most energy-dense facilities globally, with a substantial share of that energy lost as low-grade heat. Traditionally, this heat has been vented into the atmosphere — a byproduct of cooling server stacks operating around the clock.
That’s changing. In Paris, for example, Equinix’s data center is repurposing its waste heat to warm a local swimming pool and nearby residential buildings (For more info check the article here: WIRED, 2024). AI plays a critical role in this system: by predicting thermal loads, optimizing flow rates, and adapting in real-time to fluctuating energy demand, the system operates with minimal intervention. This approach not only lowers the carbon footprint of data infrastructure but also supports circular energy use at the urban level.
Manufacturing Plants: Smarter Energy Loops in Heavy Industry
In sectors like chemicals, food processing, cement, and steel, waste heat can account for 20–50% of total energy input, often at high temperatures suitable for reuse. Yet, integrating WHR into existing operations is complex — especially when each production line has its own dynamic heat profile.
AI enables manufacturing sites to build dynamic heat maps of their facilities by aggregating real-time data from SCADA, BMS, and sensor networks. These AI models identify untapped recovery potential, simulate multiple retrofit options, and recommend solutions tailored to process constraints. For example, an AI-powered ORC (Organic Rankine Cycle) model can dynamically adjust to load variations, improving energy recovery efficiency without compromising production stability (For more info check the article here: ScienceDirect, 2023).
The impact is measurable: reduced fuel consumption, lower emissions, and faster ROI on energy projects — outcomes that are increasingly tied to ESG performance metrics and regulatory compliance.
Thermoelectric Materials: Designing Smarter, from the Atom Up
While system-level optimization is critical, the materials that convert heat into usable energy are also undergoing transformation — and AI is accelerating this shift. Thermoelectric generators (TEGs), which convert temperature gradients directly into electricity, have long been limited by material inefficiencies and thermal constraints.
Now, AI is helping researchers discover and simulate new thermoelectric materials with higher conversion efficiencies and better thermal stability. Using techniques like generative machine learning and physics-informed neural networks, researchers can explore vast compositional spaces and model lattice dynamics — all in silico, before lab testing.
For instance, a recent preprint describes how AI tools trained on materials datasets identified novel bismuth-telluride-based compounds, reducing development time by orders of magnitude (For more details check the article here: arXiv, 2024).
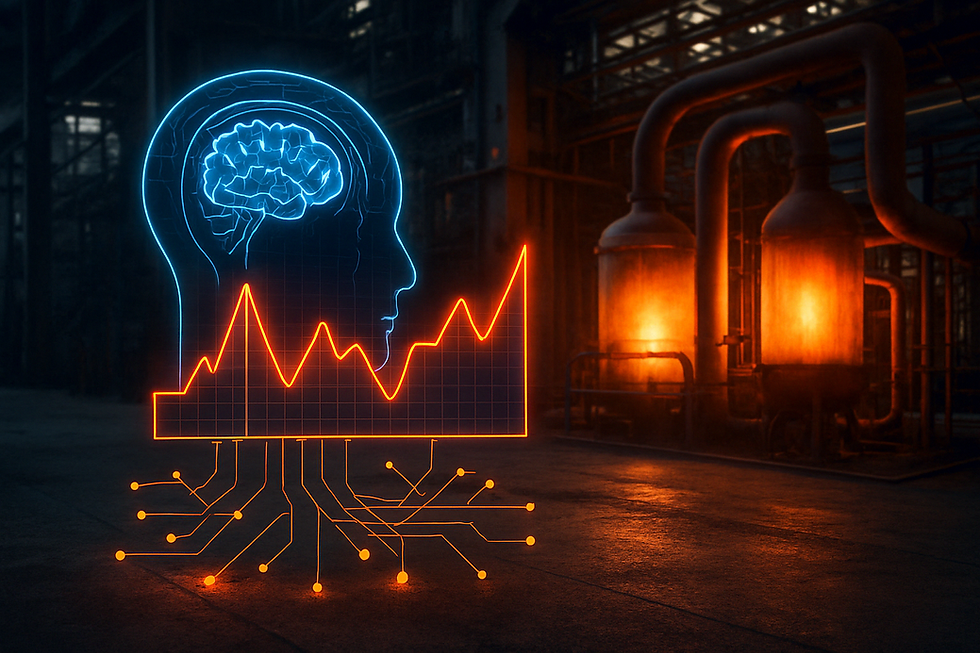
Conclusion
Harnessing AI for waste heat recovery presents a significant opportunity for industries to enhance energy efficiency, reduce costs, and contribute to environmental sustainability. By overcoming traditional challenges associated with WHR implementation, AI-driven solutions like those offered by Entropic are paving the way for a more sustainable industrial future.
Comments